In this series of interviews, three experts draw on their diverse experience with AI both in the clinic and behind the scenes.
Total running time, 2.5 hours:
Each video includes chapters which are viewable from a button at the bottom right of the player.
Interview With an Innovator, Episode 1: Steven Lin, MD
Steven Lin, MD, is the chief of general primary care and the head of technology innovation for the Division of Primary Care and Population Health at Stanford University School of Medicine. His focus is on the intersection of health services innovation, digital health and emerging technologies — specifically artificial intelligence and machine learning in healthcare. Steven is the founding director of the Stanford Healthcare AI Applied Research Team, a primary care-focused AI/ML implementation center partnered with 50 organizations across industry, academia, non-profit and government to bring leading-edge AI/ML technologies from “code to beside” in support of the Quintuple Aim. He was a James C. Puffer/American Board of Family Medicine fellow with the National Academy of Medicine and is the author of over 450 scholarly works and conference presentations.
Interview With an Innovator, Episode 2: Bob Wachter, MD
Robert M. Wachter, MD, is professor and chair of the Department of Medicine at the University of California, San Francisco (UCSF). Author of 300 articles and six books, Wachter coined the term “hospitalist” in 1996 and is often considered the “father” of the hospitalist field, the fastest growing specialty in the history of modern medicine. He is past president of the Society of Hospital Medicine, past chair of the American Board of Internal Medicine, and an elected member of the National Academy of Medicine. In 2015, Modern Healthcare magazine ranked him as the most influential physician-executive in the United States. His 2015 book, The Digital Doctor: Hope, Hype and Harm at the Dawn of Medicine’s Computer Age, was a New York Times science bestseller. In 2020-2023, Wachter’s tweets on Covid-19 were viewed more than 500 million times by 275,000 followers and served as a trusted source of information on the clinical, public health, and policy issues surrounding the pandemic. His next book, A Giant Leap: How AI Will Transform Healthcare – and What it Means for the Future, will be published in about 18 months by Portfolio, a Penguin imprint.
Interview With an Innovator, Episode 3: Katie Link
Katie Link is a product manager at NVIDIA , where she helps enable healthcare companies and researchers to solve real-world healthcare challenges with large language models (LLMs) and other advanced technologies. Prior to NVIDIA, she led healthcare and life sciences applications of artificial intelligence as a Machine Learning Engineer at Hugging Face, an open source AI startup. She is currently based in Seattle, Washington and is on leave as a medical student at the Icahn School of Medicine at Mount Sinai. While in medical school, she led artificial intelligence research at NYU Langone Hospital, creating the largest open dataset of magnetic resonance imaging (MRI) for brain metastases and developing novel deep learning algorithms for tracking cancer progression. In her spare time, she also works on AI education initiatives for medical trainees. Prior to medical school, she was an AI Resident at Google X. She holds a bachelor’s degree in Neuroscience with a minor in Computer Science from Johns Hopkins University.
Module 1: Essentials of Artificial Intelligence and Machine Learning
Author: Winston Liaw, MD, MPH
Learning Objectives
Upon completing this course, the learner should be able to:
- Define key AI/ML terms such as artificial intelligence, machine learning, and neural networks
- Identify three limitations of and risks related to AI applications
- List the steps involved with developed AI/ML tools
- Describe how AI/ML tools can be used in primary care
Module 2: Social and Ethical Implications of Artificial Intelligence and Machine Learning
Author: Winston Liaw, MD, MPH
Learning Objectives
Upon completing this course, the learner should be able to:
- Describe the mechanisms through which AI/ML tools can exacerbate health disparities
- List strategies for mitigating the negative effects of bias within AI/ML tools
- Describe ethical issues relevant to AI/ML tools
- Recognize the societal consequences of AI/ML tools
Accreditation
American Academy of Family Physicians (AAFP) CME Credit
The AAFP has reviewed Artificial Intelligence and Machine learning for Primary Care, and deemed it acceptable for AAFP credit. Term of approval is from 12/13/2024 to 12/12/2025. Physicians should claim only the credit commensurate with the extent of their participation in the activity.
This session, Module 1: Artificial Intelligence / Machine Learning (AI/ML) Essentials, is approved for up to 1.0 Enduring Materials, Self-Study AAFP Prescribed credit.
This session, Module 2: Social and Ethical Implications of AI/ML, is approved for up to 1.0 Enduring Materials, Self-Study AAFP Prescribed credit.
AMA/AAFP Equivalency: AAFP Prescribed credit is accepted by the American Medical Association as equivalent to AMA PRA Category 1 credit(s)™ toward the AMA Physician's Recognition Award. When applying for the AMA PRA, Prescribed credit earned must be reported as Prescribed, not as Category 1.
Certificate of Participation
Upon completing the activity requirements, learners will receive a certificate of participation. This certificate can be submitted to licensing boards or associations not preapproved for credits. For credit types not listed above, participants should follow the application process outlined by the specific organization to obtain recognition.
Release Date: December 13, 2024Expiration Date: December 12, 2025
Target Audience: This activity is tailored to meet the educational needs of medical students, primary care residents, and practicing primary care physicians in academic and community settings.
Mitigation of Relevant Financial Relationships
The AAFP, in collaboration with STFM and ABFM, strictly adheres to the Accreditation Council for Continuing Medical Education's (ACCME) Standards for Integrity and Independence in Accredited Continuing Education. In alignment with these standards, all individuals involved in the development and oversight of educational content are required to disclose any financial relationships they have had with ACCME-defined ineligible companies within the past 24 months. Ineligible companies, as defined by the ACCME, are entities primarily engaged in producing, marketing, selling, reselling, or distributing healthcare products used by or on patients. Disclosures must encompass all financial relationships, regardless of their size or perceived relevance to the educational content.
The AAFP and its collaborators are committed to ensuring that all educational activities remain free from commercial bias. For this activity, no relevant financial relationships with ineligible companies have been disclosed by any individual involved in content development or oversight.
The planners, content developers, and peer reviewers from AAFP, the accredited provider, along with its collaborating partners STFM and ABFM, have no relevant financial relationships to disclose with any ACCME-defined ineligible companies.
Faculty Member Information
|
Role in Activity
|
Name of Ineligible Company(s) /
Nature of Relationship(s)
|
Winston Liaw, MD, MPH
Chair, Health Systems and Population Health Sciences
Tilman J. Fertitta Family College of Medicine
University of Houston
|
Course Director; Curriculum Lead
|
Dr. Liaw has no relevant
financial relationships with ineligible companies to disclose.
|
Cornelius A. James, MDAssistant Professor of Internal Medicine, Assistant Professor of Pediatrics,
Assistant Professor of Learning Health Sciences
University of Michigan Medical School
|
Faculty; Curriculum Author
|
Dr. James has no relevant
financial relationships with ineligible companies to disclose.
|
Ioannis Kakadiaris, PhD
Professor, Health Systems and Population Health Sciences
Tilman J. Fertitta Family College of Medicine
Founder and Director, Computational Biomedicine Lab
Hugh Roy and Lillie Cranz Cullen University Professor of Computer Science, Electrical & Computer Engineering, and Biomedical Engineering
University of Houston
|
Curriculum Author
|
Dr. Kakadiaris has no relevant financial relationships with ineligible companies to disclose.
|
Jacqueline K. Kueper, PhD
Senior Research Epidemiologist, Digital Trials Center
Scripps Research Institute
|
Curriculum Author
|
Dr. Kueper has no relevant
financial relationships with ineligible companies to disclose.
|
Vasiliki (Vaso) Rahimzadeh, PhD
Assistant Professor, Center for Medical Ethics & Health Policy - Research
Baylor College of Medicine
|
Curriculum Author and AI Ethics Reviewer
|
Dr. Rahimzadeh has no relevant financial relationships with ineligible companies to disclose.
|
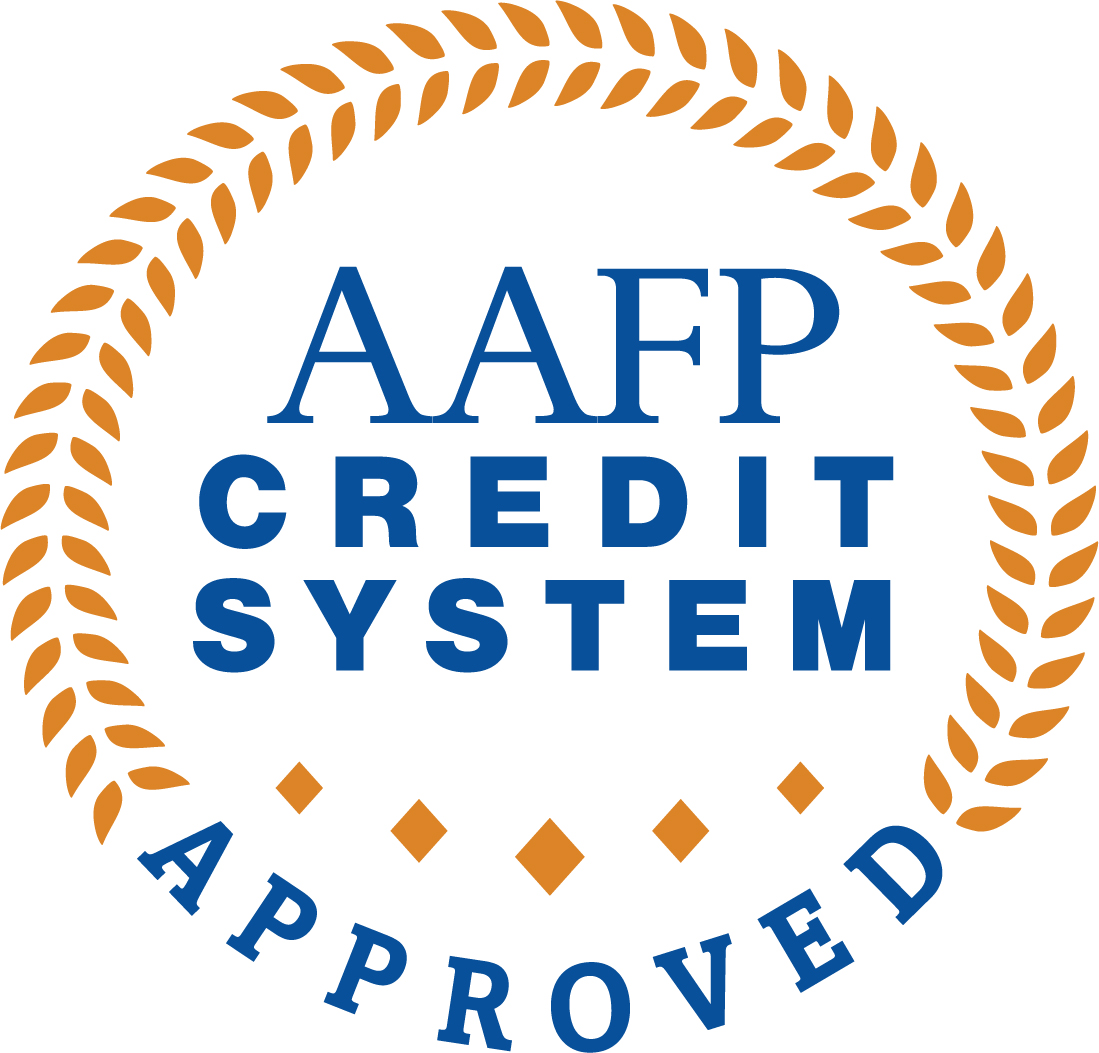
In the second series of interviews, three more experts draw on their experience with AI both in the clinic and behind the scenes.
Total running time, 2 hours:
Each video includes chapters which are viewable from a button at the bottom right of the player.
Interview With an Innovator, Episode 4: Nipa Shah, MD
Nipa Shah, MD, shares her experiences integrating AI into the clinic, emphasizing the importance of equitable access, reducing clinician burnout, and addressing algorithmic bias. Dr. Shah shows how AI can enhance patient care, remove language barriers, and provided additional layers of operational efficiency.
Interview With an Innovator, Episode 5: Judy Gichoya, MD, MS
Judy Gichoya, MD, MS, addresses the important role that data plays in both clinical use and research that involves AI. She shares some of the challenges of algorithmic bias, the importance of data diversity, and the need for clinician involvement in AI development. As she continues discussing ethical considerations, Dr Gichoya provides insights into data security and secure data storage.
Interview With an Innovator, Episode 6: Jenna Wiens, PhD
Jenna Wiens, PhD talks about the evolving role of predictive AI in healthcare, highlighting its potential to personalize care, enhance clinical workflows, and improve patient outcomes. Dr. Wiens also discusses challenges such as bias mitigation, model interpretability, and equitable access to AI tools. She shares insights from her research on predictive analytics and offers practical advice for integrating AI into clinical practice while maintaining trust and prioritizing safety.
The Artificial Intelligence and Machine Learning for Primary Care (AiM-PC) Curriculum for medical students, primary care residents, and practicing primary care clinicians was developed in conjunction with the Society of Teachers of Family Medicine (STFM) and American Board of Family Medicine, funded by the Gordon and Betty Moore Foundation, and supported by an advisory committee. Our team consists of educators with expertise in primary care, computer science, instructional design, and ethics. By harnessing these diverse perspectives, AiM-PC will equip learners with the skills needed to be engaged stakeholders, use AI/ML in their practice, and ensure responsible and ethical use of AI/ML.
The modules in this course include:
- Module 1: AI/ML Essentials
- Module 2: Social and Ethical Implications of AI/ML
- Module 3: Evidence-Based Evaluation of AI/ML-Based Tools
- Module 4: AI/ML-Enhanced Clinical Encounters
- Module 5: Integrating AI/ML into the Clinic
While these modules are numbered, you can take them in any order you like. We recommend you begin with the first module.
Our goals for this curriculum are:
- To equip learners with the skills necessary to be engaged stakeholders in a clinical world that includes AI/ML
- To develop and disseminate innovative health care AI/ML curricula to prepare the primary care workforce
- To create efficient and effective AI/ML curricula that can be applied across various primary care settings
- To prepare learners in primary care settings to appraise and apply literature and outputs in their clinical practice
- To ensure that all patients benefit from AI/ML-augmented clinical care